Top 10 Use Cases of Retrieval-Augmented Generation (RAG) - P1
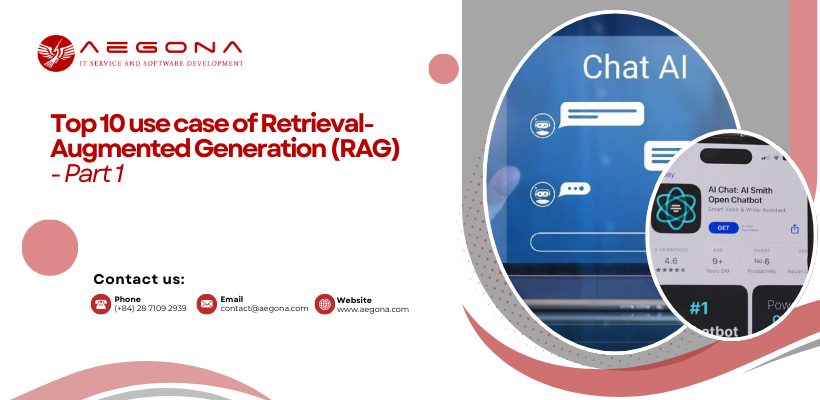
Today, Retrieval-Augmented Generation (RAG) technology enables AI systems to process queries and synthesize information from multiple sources to produce accurate and timely responses. In this article, let's explore five use cases of RAG is applied, such as finance, legal, healthcare, agriculture, and pharmaceuticals.
Use cases of retrieval-augmented generation technology
1. Application of RAG technology in Finance
In the financial sector, RAG has broad potential to address the limitations of large language models (LLMs). According to FINANCEBENCH, a valuable resource for researchers and developers working on LLMs in finance, all tested LLMs exhibit weaknesses like hallucinations (generating false information), limiting their suitability for financial applications.
To address these challenges, experts have called for further research to develop LLMs that can better handle financial question-answering issues. These challenges include:
- Specialized knowledge: LLMs need to understand financial terminology, concepts, and regulations.
- Access to updated information: Financial data changes frequently, so LLMs need real-time or near-real-time data access.
- Numerical reasoning: LLMs often struggle with arithmetic calculations.
- Handling structured and unstructured data: Financial information can be presented as tables, charts, and text, requiring LLMs to process all these formats.
- Reasoning with multiple sources: LLMs must analyze information from various sources to draw conclusions.
By combining the content generation capability of LLMs with the ability to retrieve information from vast data sources, RAG can provide more accurate, up-to-date, and reliable answers.

2. Application of RAG Technology in Legal – Law
A prime example of RAG in the legal field is DISC-LawLLM, an intelligent legal system that uses LLMs to provide a variety of legal services. DISC-LawLLM applies RAG by combining two main steps:
- Supervised Fine-Tuning:
- Build a DISC-Law-SFT dataset: This dataset includes legal tasks such as extracting legal elements, predicting verdicts, and answering legal questions, created with the help of a general-purpose LLM.
- Fine-tune on a pre-trained LLM to equip it with legal reasoning and judicial behavior.
- Enhanced Retrieval:
- A comprehensive knowledge base containing Chinese laws is constructed.
- A retrieval module pulls relevant legal documents from the knowledge base based on user input.
- The model is further trained with a specific subset of the SFT dataset to leverage retrieved information for more reliable responses.
DISC-LawLLM has been praised for its understanding of legal matters and reasoning abilities through subjective and objective evaluations. This demonstrates that RAG is a promising technique for building intelligent legal systems that can provide diverse legal services with high accuracy and reliability.
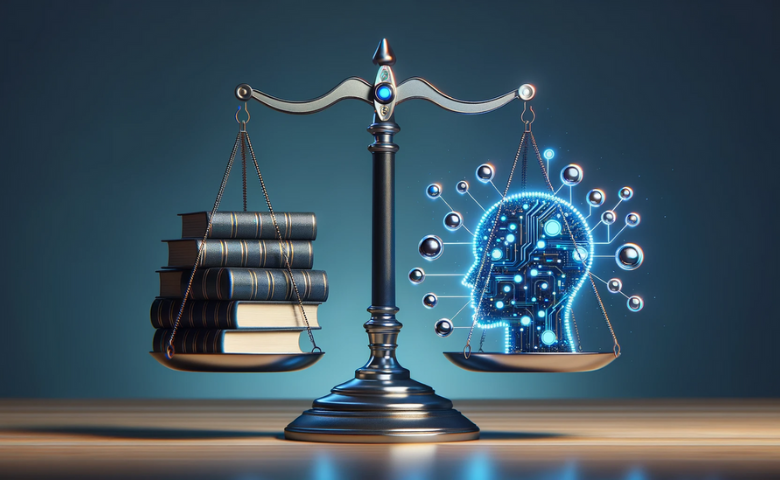
3. Application of RAG Technology in Healthcare
A recent study demonstrated the effectiveness of RAG in improving the ability of large language models to generate accurate SQL queries for extracting information from Electronic Health Records (EHR) and claims data. The authors used RAG combined with medical coding and various prompting methods to enhance the performance of LLMs in answering epidemiological questions.
The study's results show that RAG can help researchers and healthcare organizations analyze data more effectively to answer epidemiological questions and make data-driven decisions. However, the study also pointed out that the current dataset is limited in scale and focuses on epidemiological questions. Thus, further research and development are needed to expand RAG's scope in healthcare and address challenges related to using electronic health data.

4. Application of RAG Technology in Agriculture
In agriculture, RAG is used to improve the performance of large language models in answering agricultural-related questions. A recent study compared the effectiveness of RAG with Fine-Tuning in integrating specialized knowledge into LLMs for the agriculture sector. The results indicate that both RAG and Fine-Tuning can improve LLM performance, but each has its advantages and disadvantages:
RAG: Effective when dealing with context-related data, leading to concise and accurate answers.
Fine-Tuning: Provides more specific and accurate answers by teaching the model new skills related to agriculture. However, the initial cost is higher due to the extensive work required to fine-tune the model to new data.
The study also found that GPT-4 generally outperforms other models (such as Llama2-13B and Vicuna) but at a higher inference cost.
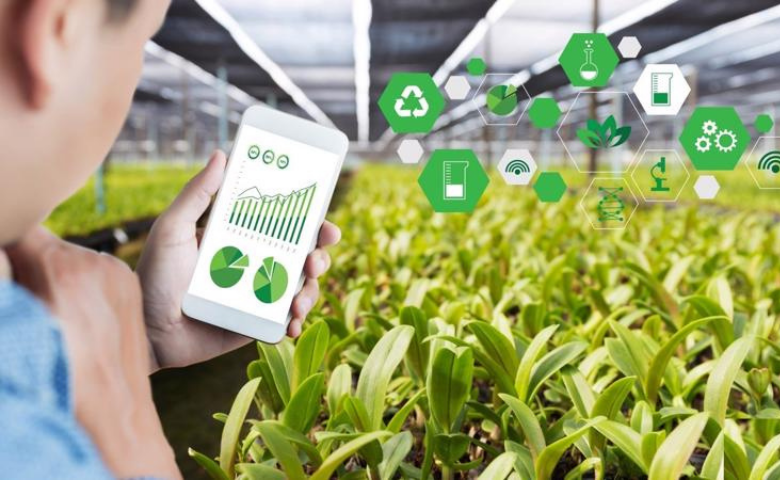
5. Application of RAG Technology in Pharmaceuticals
In the pharmaceutical sector, RAG can be used to improve regulatory compliance performance. A recent study introduced the QA-RAG model, an approach that integrates RAG and generative AI to assist in searching complex regulatory information in the pharmaceutical industry. QA-RAG uses a fine-tuned LLM to generate relevant hypothetical answers to user queries, then uses both the query and the hypothetical answer to search for related documents.
The study results show that QA-RAG outperforms other methods in both the accuracy of document retrieval and the accuracy of generated answers. This indicates the effectiveness of using fine-tuned LLMs combined with hypothetical answers to enhance search accuracy in the pharmaceutical field.

>> Read more:
- Sales Technology Trends 2024: Integrating AI into CRM software
- How Much Is The Cost Of Mobile App Development In Vietnam?
II. Aegona – AI Development Company Specializing in RAG Applications
Aegona is a professional software company specializing in software development and integrating AI solutions into modern systems. With extensive experience in technology, Aegona not only provides AI development solutions but also focuses on applying Retrieval-Augmented Generation techniques to help businesses solve complex problems.
The AI solutions offered by Aegona include:
- AI development from leading platforms such as OpenAI, TensorFlow, Microsoft Azure AI, Vertex AI, Keras, H2O.ai, Amazon SageMaker, etc.
- AI-integrated system software outsourcing: Helping businesses optimize processes and improve decision-making capabilities.
- Developing mobile apps with intelligent virtual assistants: Enhancing user experience and interaction.
- Designing websites integrated with smart chatbots: Automatically providing information and answers to customers, reducing costs, and increasing service efficiency.
III. Final Thought
Retrieval-Augmented Generation technology and modern AI solutions are opening new opportunities for businesses, improving efficiency, and automating processes effectively. Stay tuned for the next part, where we will explore five more sectors utilizing RAG.
Which sector interests you the most? Feel free to share with us via email at [email protected] or contact number +84 914 528 869 (Whatsapp) for advice and support for the success of the project.
AEGONA LTD - IT SERVICE & SOFTWARE DEVELOPMENT
Email: contact@aegona.com
Phone: Office: (+84) 28 7109 2939. Hotline: (+84) 91 451 8869 | (+84) 83 940 5469
WhatsApp: (+84) 91 451 8869
Website: www.aegona.com, www.aegona.vn
Address: Orbital Building (QTSC Building 9), Quang Trung Software City, District 12, Ho Chi Minh city, Vietnam.
related post
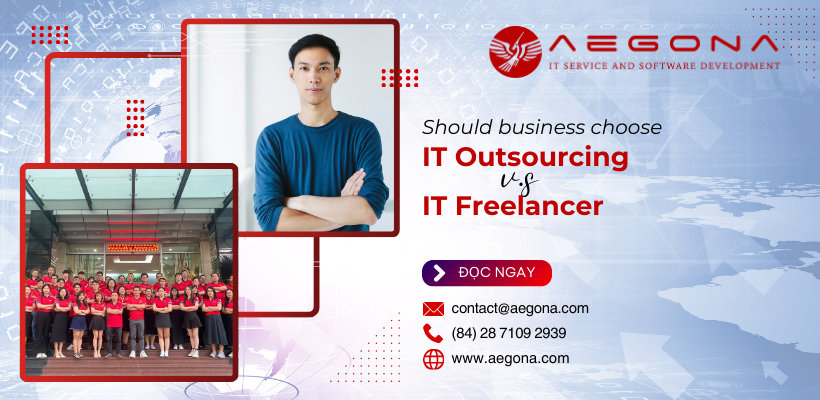
Businesses, regardless of whether they operate in the tech industry, are increasingly turning to IT outsourcing to reduce operational costs and improve efficiency. However, many are still unsure whether to go with an IT outsourcing service or hire a freelancer. Which is the best choice for your business? Let’s explore the answer with Aegona in the article below!
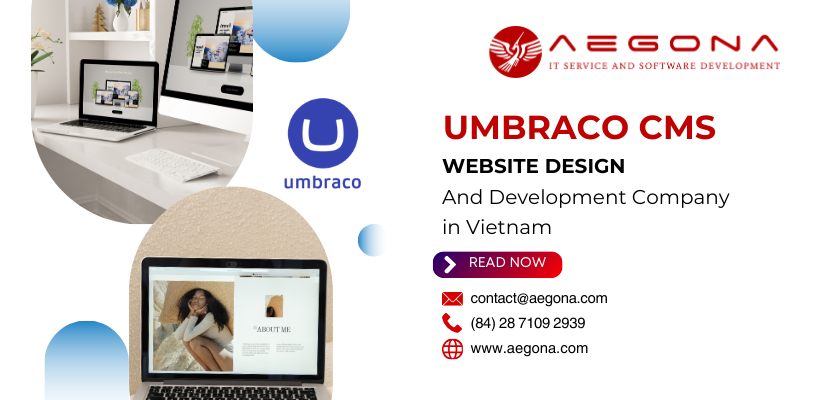
Umbraco CMS (.NET) is one of the most optimized content management platforms compared to other popular CMS platforms on the market today. However, in Vietnam, Umbraco is still relatively underutilized and not commonly chosen for CMS web development.
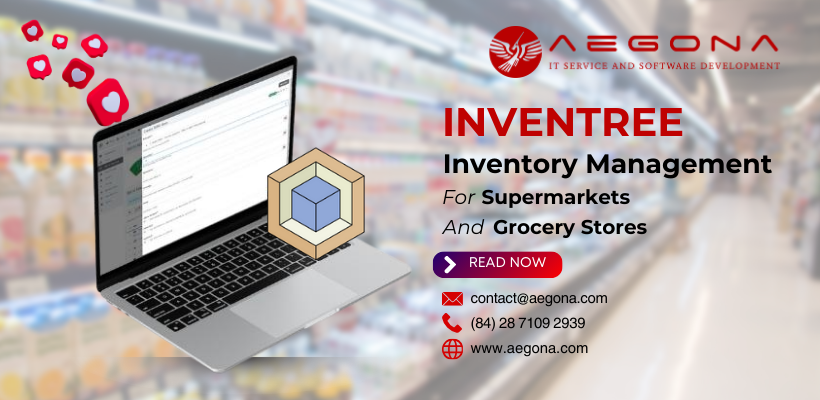
Inventory management effectively is essential for the success of supermarkets and grocery stores. This guide outlines how InvenTree inventory software streamlines each stage of the process, from warehouse intake to analytics and reporting.
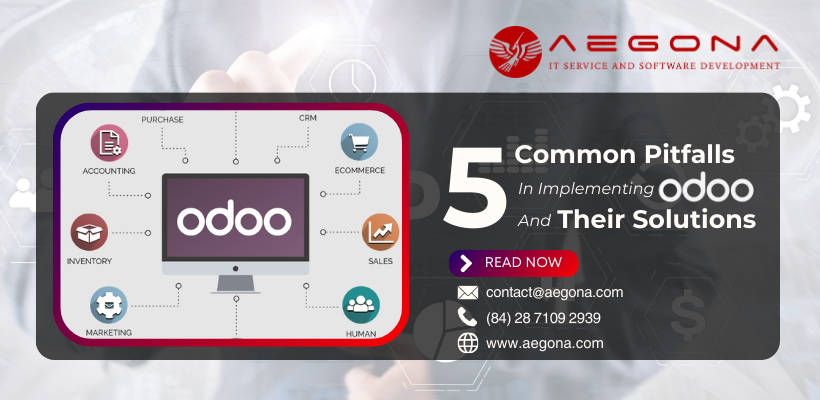
Implementing Odoo ERP brings numerous benefits to business operations by optimizing processes and increasing efficiency. However, an incomplete implementation plan can lead to costly mistakes and delays.
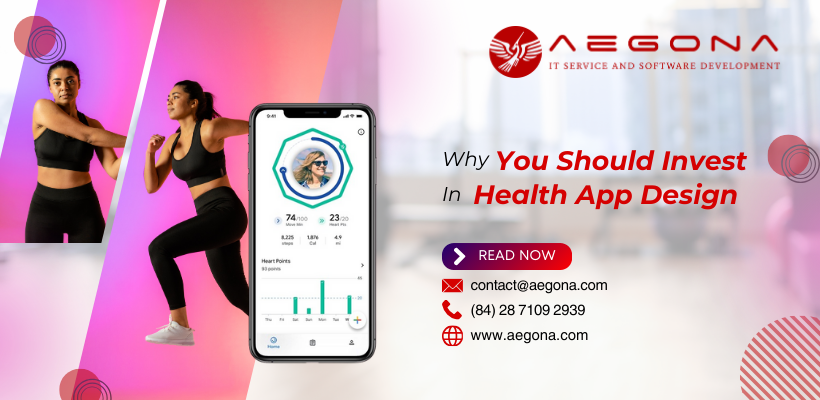
Among them, health app design is becoming a promising startup trend. Applications like Google Fit, Samsung Health, and Doctor Anywhere have attracted millions of users thanks to their comprehensive support, convenience, and personalized experiences. So why should businesses invest in health app design starting today? Let’s explore some standout benefits with AEGONA.
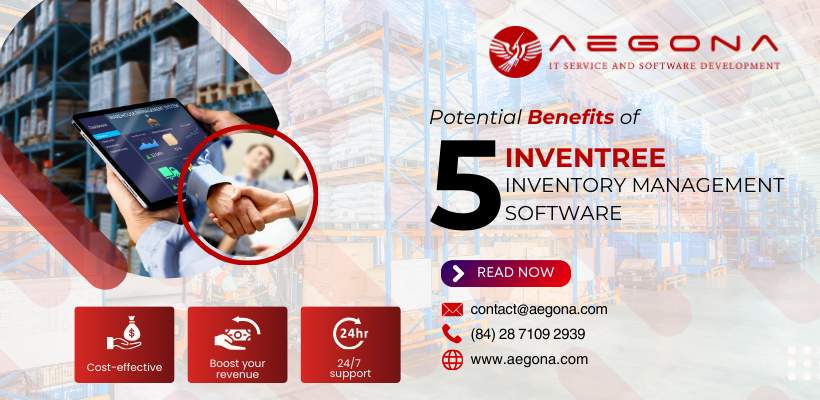
Optimizing inventory management processes is a key factor in helping businesses improve operational efficiency and minimize costs. Inventree inventory management software is an all-in-one solution trusted by many businesses. This software offers practical benefits, making inventory management easier and more efficient. Let’s explore the standout advantages of Inventree with AEGONA in the article below.
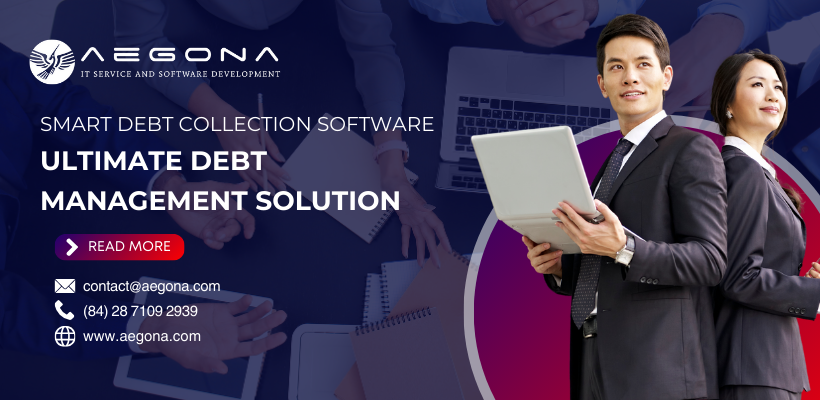
Debt management software is a modern solution that enables businesses to efficiently manage and process outstanding debts. Aegona pioneers in implementing, integrating, and customizing professional and effective debt management software. With features such as automated reminders, payment tracking, and detailed reporting, this software helps businesses enhance financial control and minimize bad debt risks.
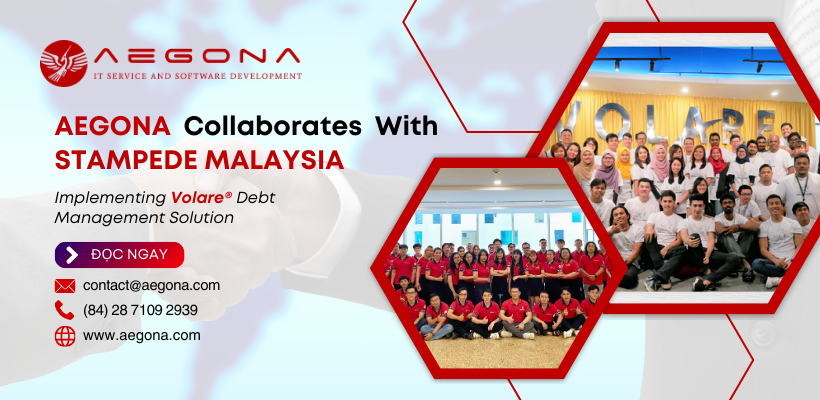
In the rapidly evolving financial market, the adoption of technology to optimize financial management processes is crucial for businesses to maintain competitiveness. AEGONA has partnered with Stampede Malaysia to implement Volare®, a comprehensive financial management system designed to optimize debt collection and accounts receivable processes for businesses.
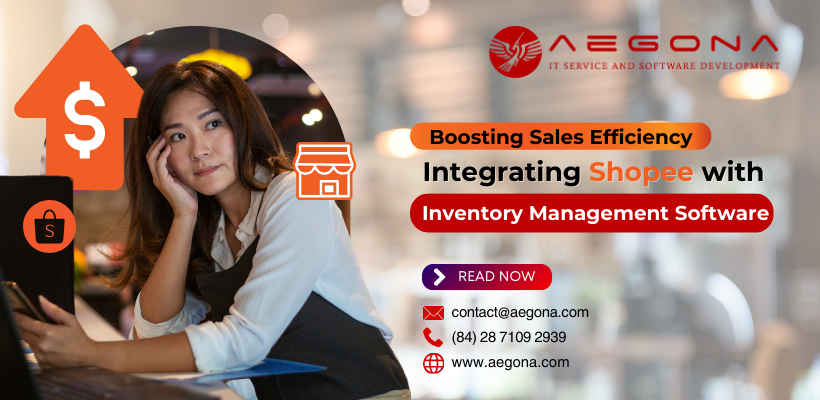
In 2025, the e-commerce platform Shopee is increasing its service fees, posing significant challenges for sellers. How can businesses adapt and optimize their operations under these new conditions? Many shop owners are turning to Aegona’s inventory management software as an effective solution to streamline stock control, enhance operations, and automate sales processes.
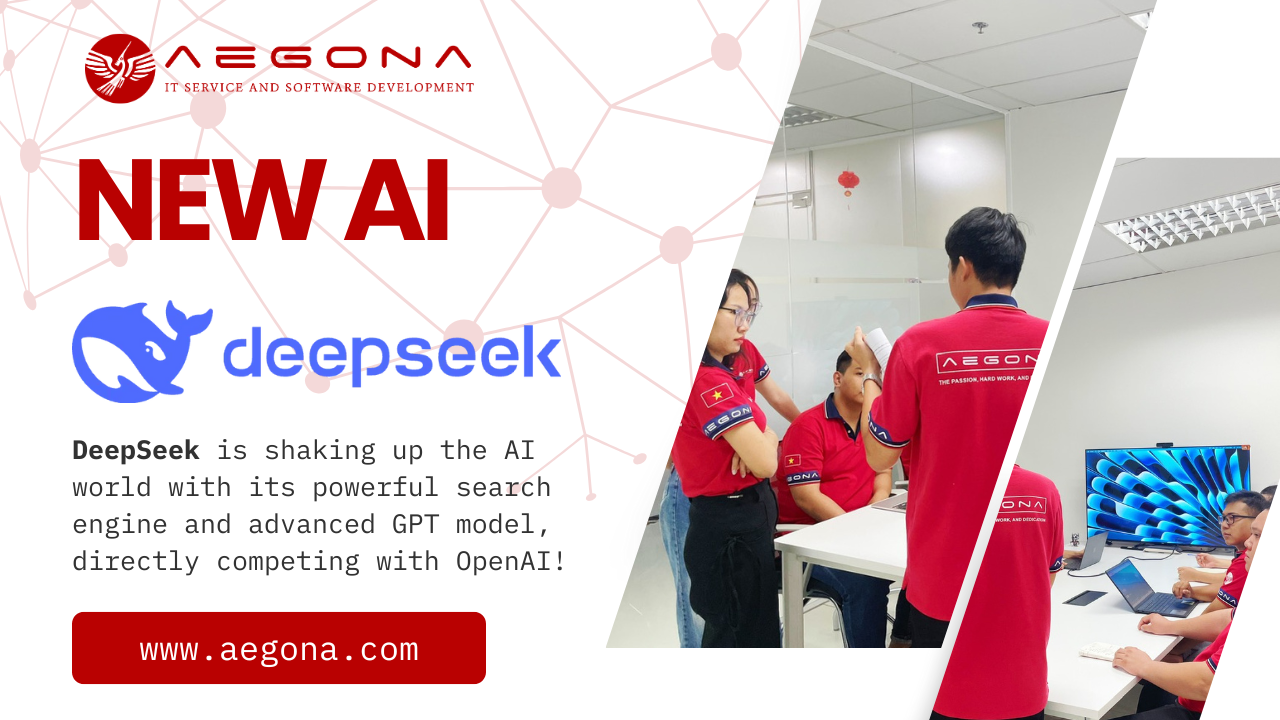
Discover DeepSeek, the advanced AI assistant revolutionizing technology. Learn how DeepSeek can enhance productivity, automate tasks, and deliver intelligent solutions
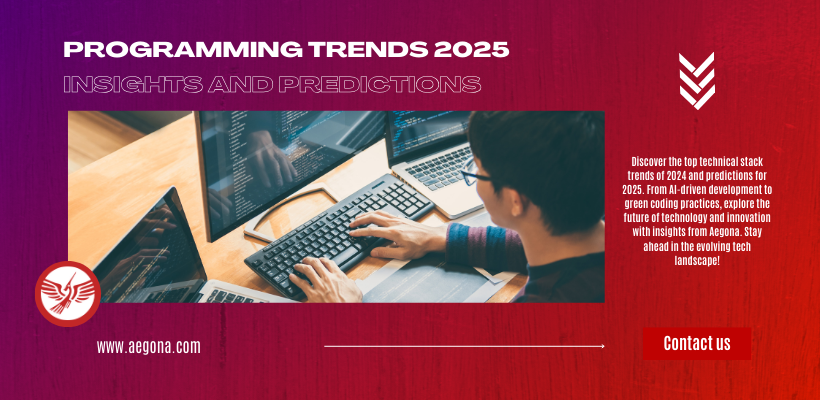
In the ever-evolving world of technology, programming trends play a critical role in shaping the future of innovation. As we bid farewell to 2024, it’s time to look back at the trends that defined the year and anticipate what’s ahead in 2025. Let’s explore the most popular programming trends of 2024 and the emerging trends that will dominate the tech landscape.
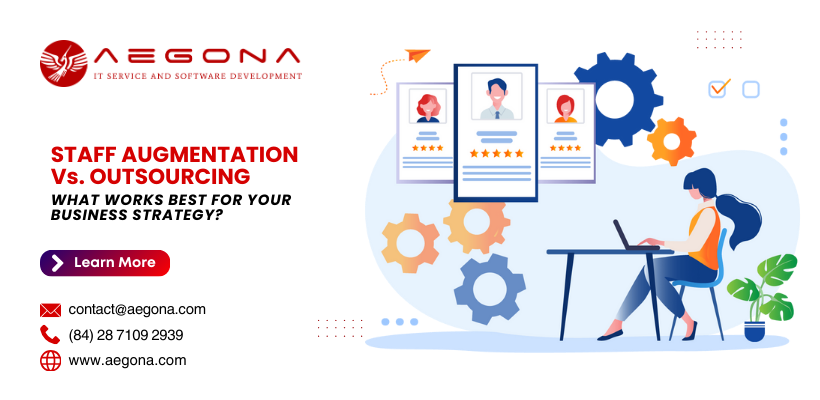
Discover the differences between staff augmentation and project outsourcing. Learn which model best suits your business goals and IT needs.
![Top 5 Open-Source Document Management Systems [Update 2025]](/sites/default/files/2024-12/Top-5-open-source-document-management-systems.png)
Looking for the best open-source and self-hosted document management software? Explore the top five open-source solutions that provide free document management.
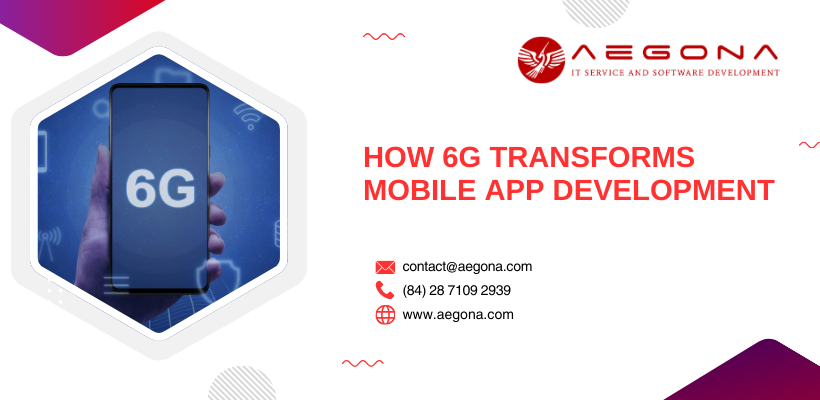
6G empowers app developers and businesses to push boundaries like never before. Let’s explore how this next-generation network is transforming the app development landscape.
